Remote Sensing of Mine Contaminants using Satellite Products
- john06025
- Mar 27
- 3 min read
Updated: Mar 28
Mining operations, whether for coal or minerals, release various contaminants into the environment. These may include coal dust, sulfur compounds, acid mine drainage (AMD), and heavy metals such as arsenic, mercury, cadmium, selenium, and lead. Historic abandoned mines, where rehabilitation may be incomplete and stakeholders are unclear, are of particular interest.
Consider, for example, Mt. Morgan Mine in Queensland, which operated from the late 1800s to the early 1990s, producing gold, copper, and silver. Following its closure, contaminated runoff from the mine site reportedly impacted nearby water sources, including the Boyne River. In response, in 2009 the Queensland government announced a remediation plan [1].
The scale of abandoned mines in Australia is significant. A study by Monash University identified more than 80,000 inactive mines [2]. Of these, 68% were classified by the authors as "neglected", while only 4% have been rehabilitated. New South Wales contained over 26,000 inactive mines, and Victoria over 18,000.
Here we consider some interesting work, at the development stage, regarding remote sensing of mine contaminants. Whilst drone-based hyper-spectral analysis is more well understood, it relies on site visits; we are primarily interested in the use of satellite products and the potential for developing a screening modality. Considering the huge area, which Australian abandoned mines inhabit, the benefit of being able to risk assess these sites remotely, would be significant.
The study of interest was a "kick start activity" project called RiPolMin, conducted in conjunction with ESA and various other UK partners [3]. The project leveraged multi-spectral Sentinel-2 satellite products, which are open source, have global coverage, 10-20m spatial resolution, and a 5 day revisit time. The authors used a Sentinel-2 "screening tool to locate problem areas at regional scale". They then used drones for more granular analysis of the target sites (Fig.1).

Fig.1 Example mine with contaminant heatmap. Top: Sentinel-2 (presumed). Bottom: drone RGB and 3D model [3].
Regarding he development of a satellite screening tool, this authors do not explicitly discuss their methodology, but we can infer it, by considering related modalities. i.e. a training set is developed, where ground truth labels are obtained from site surveys of contaminated mines. Corresponding cloud-free Sentinel-2 products are obtained. Pixel-wise multi-spectral modelling of the Sentinel-2 products can then be carried out, using supervised modelling modalities. This process is related to the remote sensing of soil organic carbon (see Castaldi et al. 2019 [4], and our related blog post), and also the remote sensing of vegetation (pixel-wise supervised modelling of multi-spectral products).
Example hyper-spectral mine surveys are shown below (Fig.2). Obtaining sufficiently rich ground truth data is likely the primary development challenge, because of the required investment in site surveys.
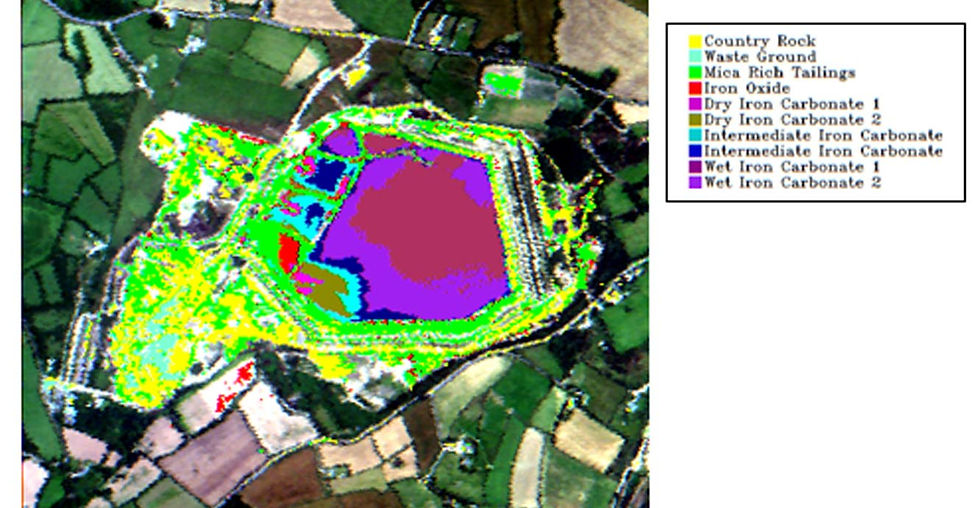
![Fig.2 Top: mine waste map of the tailings dam at Wheal Jane mine, Cornwall [5]. Bottom Left: example mine waste mapping, with red pixels indicating the most zinc- and lead- rich tailings. Bottom Right: sampling sites for lab analysis [5].](https://static.wixstatic.com/media/86b4fe_d69c2322c5804ddd9965f04a7a1bedbe~mv2.jpg/v1/fill/w_980,h_508,al_c,q_85,usm_0.66_1.00_0.01,enc_avif,quality_auto/86b4fe_d69c2322c5804ddd9965f04a7a1bedbe~mv2.jpg)
References
[1] Queensland Government (2019): "Mt. Morgan Mine Rehabilitation." Department of Environment and Science, Queensland Government. https://www.qld.gov.au/environment/land/management/abandoned-mines/remediation-projects/mount-morgan
[2] Unearthing a gold standard for rehabilitating 80,000 mines in Australia, Monash University. https://www.monash.edu/news/articles/unearthing-a-gold-standard-for-rehabilitating-80%2C000-mines-in-australia?utm_source=chatgpt.com
[3] ESA outline of the RiPolMin project. https://business.esa.int/projects/ripolmin
[4] Castaldi, F., Hueni, A., Chabrillat, S., Ward, K., Buttafuoco, G., Bomans, B., Vreys, K., Brell, M. and van Wesemael, B., 2019. Evaluating the capability of the Sentinel 2 data for soil organic carbon prediction in croplands. ISPRS Journal of Photogrammetry and Remote Sensing, 147, pp.267-282.
[5] Hyperspectral research, MINEO project, British Geological Survey. https://www.bgs.ac.uk/geology-projects/geodesy/hyperspectral-research/
Comments